As you know, I’m a techno-optimist. I particularly love AI and have huge hopes that it will dramatically improve our lives. But I am also concerned about its risks, especially:
- Will it kill us all?
- Will there be a dramatic transition where lots of people lose their jobs?
- If any of the above are true, how fast will it take over?
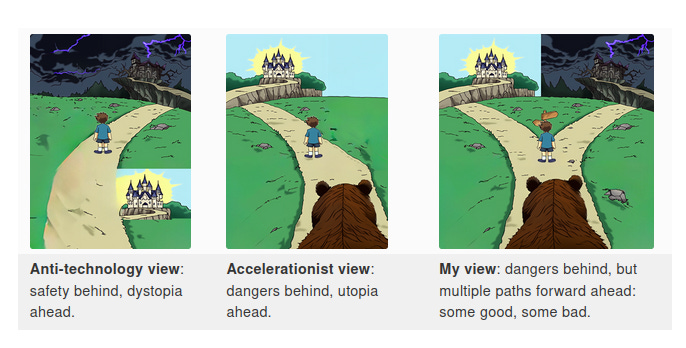
This is important enough to deserve its own update every quarter. Here we go.
Why I Think We Will Reach AGI Soon
“AGI by 2027 is strikingly plausible. GPT-2 to GPT-4 took us from ~preschooler to ~smart high-schooler abilities in 4 years. We should expect another preschooler-to-high-schooler-sized qualitative jump by 2027.” – Leopold Aschenbrenner.
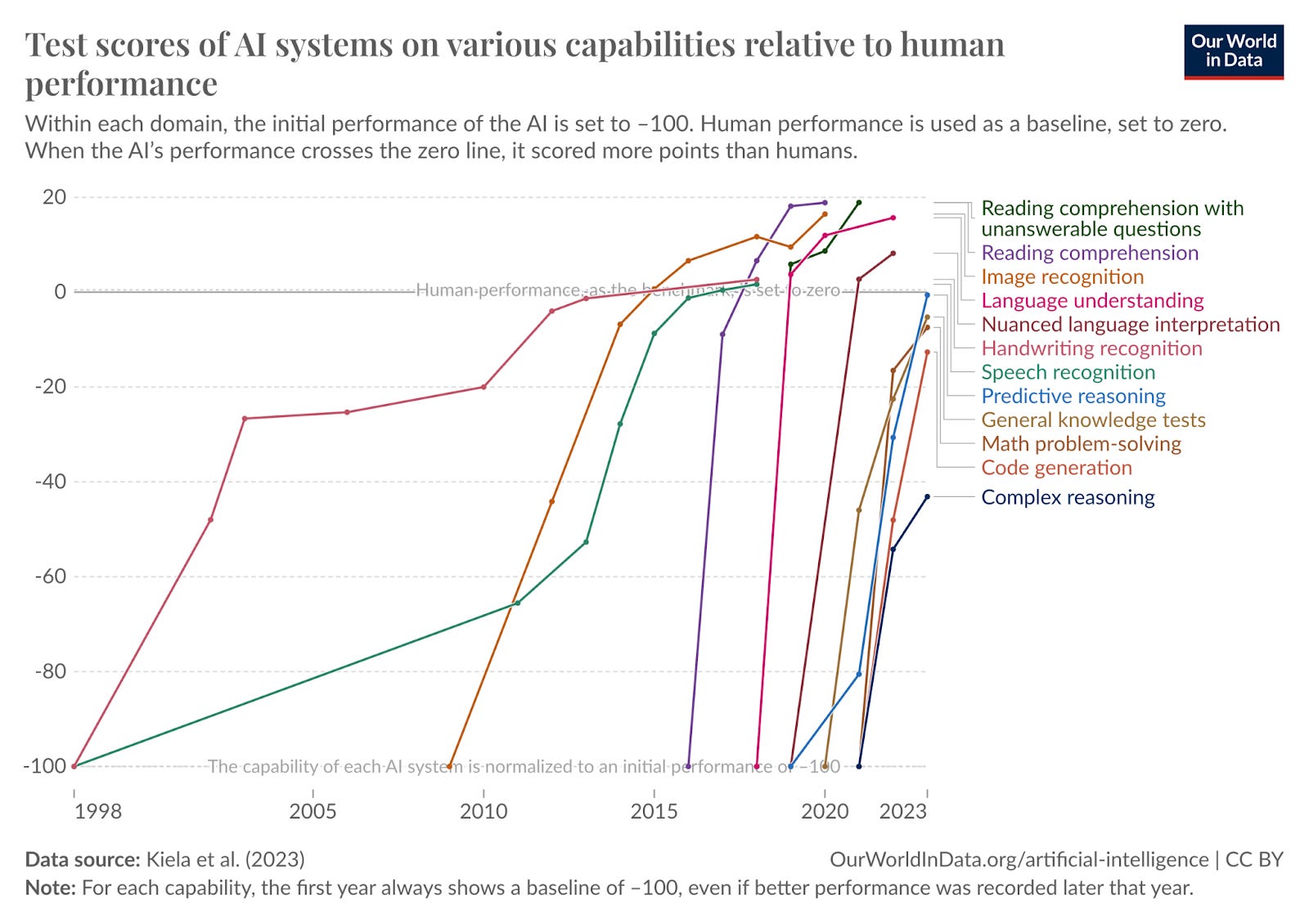
In How Fast Will AI Automation Arrive?, I explained why I think AGI (Artificial General Intelligence) is imminent. The gist of the idea is that intelligence depends on:
- Investment
- Processing power
- Quality of algorithms
- Data
Are all of these growing in a way that the singularity will arrive soon?
1. Investment
Now we have plenty of it. We used to need millions to train a model. Then hundreds of millions. Then billions. Then tens of billions. Now trillions. Investors see the opportunity, and they’re willing to put in the money we need.
2. Processing Power
Processing power is always a constraint, but it has doubled every two years for over 120 years, and it doesn’t look like it will slow down, so we can assume it will continue.
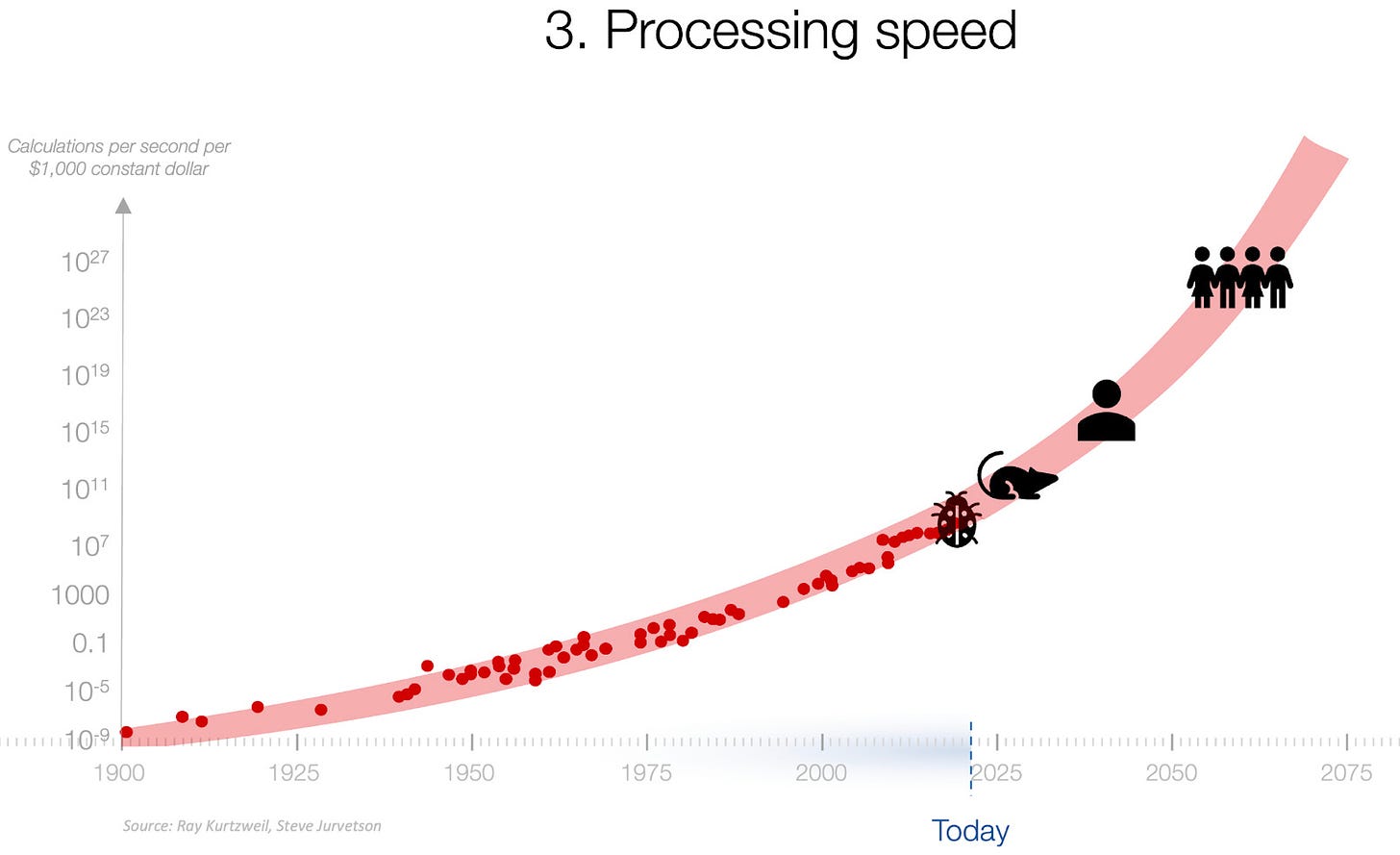
This is a zoom in on computing for the last few years in AI (logarithmic scale):
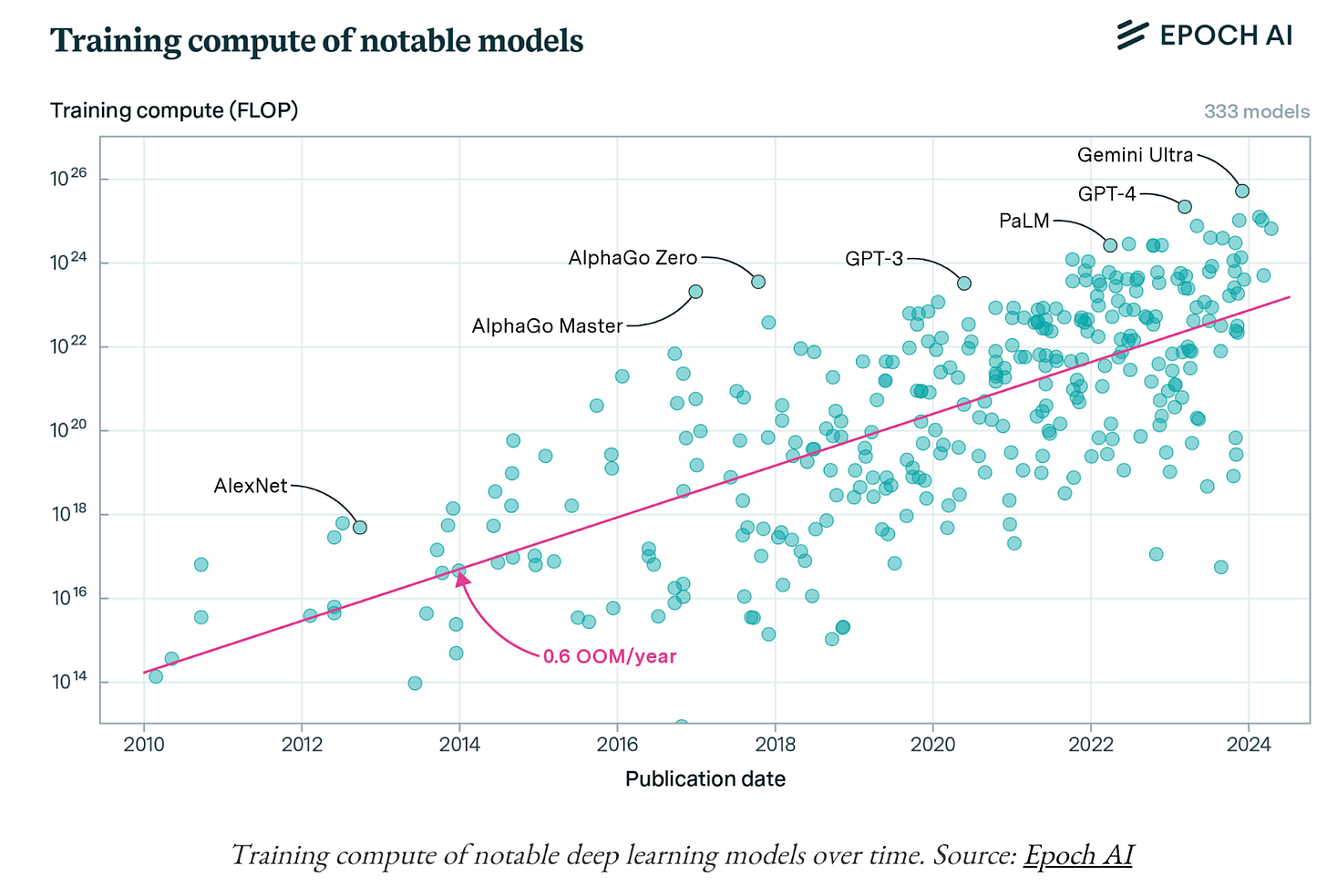
Today, GPT-4 is at the computing level of a smart high-schooler. If you forecast that a few years ahead, you reach AI researcher level pretty soon—a point at which AI can improve itself alone and achieve superintelligence quickly.
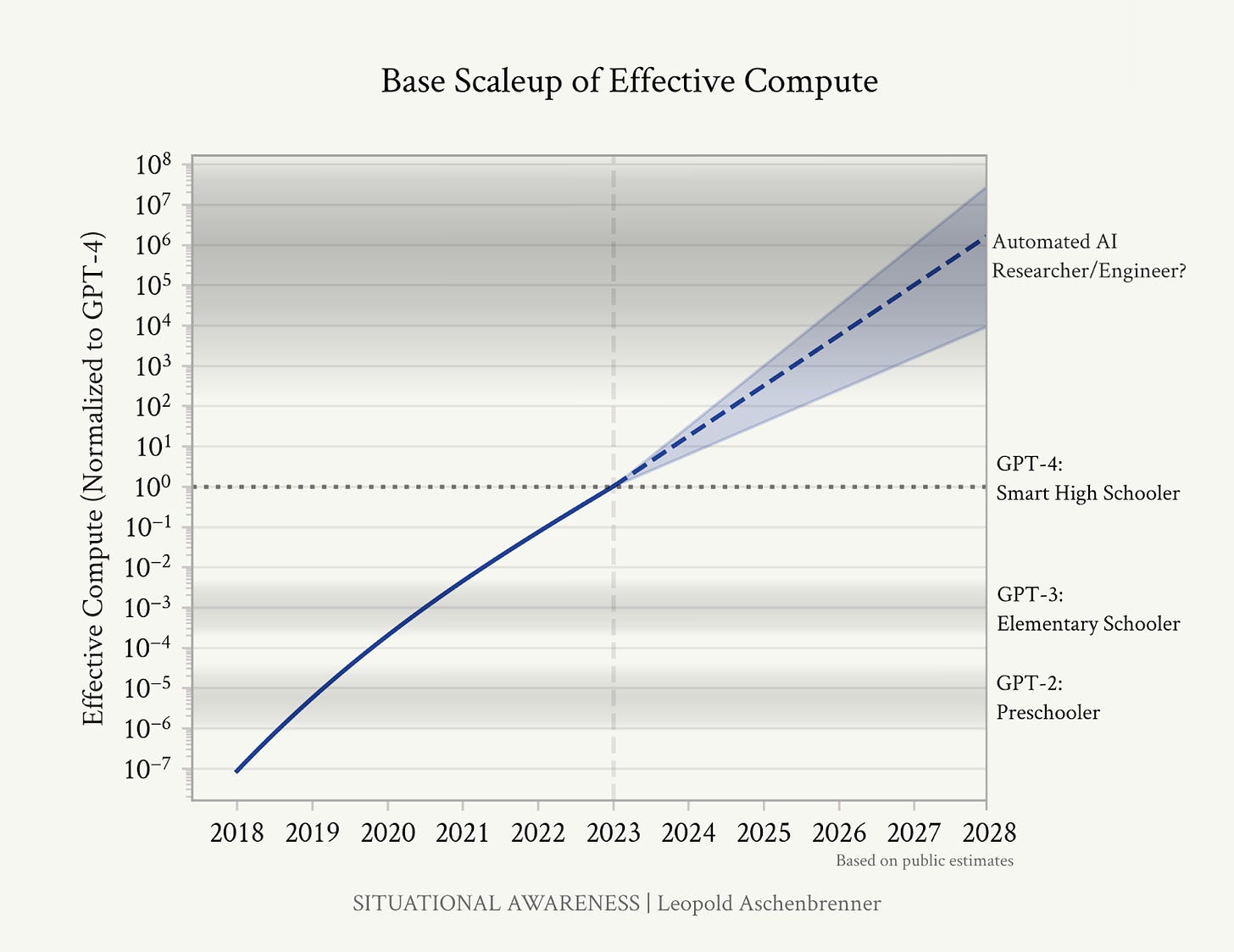
3. Algorithms
I’ve always been skeptical that huge breakthroughs in raw algorithms were important to reach AGI, because it looks like our neurons aren’t very different from those of other animals, and that our brains are basically just monkey brains, except with more neurons.
This paper, from a couple of months ago, suggests that what makes us unique is simply that we have more capacity to process information—quantity, not quality.
Here’s another take, based on this paper:
“These people developed an architecture very different from Transformers [the standard infrastructure for AIs like ChatGPT] called BiGS, spent months and months optimizing it and training different configurations, only to discover that at the same parameter count, a wildly different architecture produces identical performance to transformers.“
There could be some magical algorithm that is completely different and makes Large Language Models (LLMs) work really well. But if that’s the case, how likely would it be that a wildly different approach yields the same performance when it has the same power? That leads me to think that parameters (and hence processing) matters much more than anything else, and the moment we get close to the processing of a human brain, AGIs will be around the corner.
Experts are consistently proven wrong when they bet on the need for algorithms and against compute. For example, these experts predicted that passing the MATH benchmark would require much better algorithms, so there would be minimal progress in the coming years. Then in one year, performance went from ~5% to 50% accuracy. MATH is now basically solved, with recent performance over 90%.
It doesn’t look like we need to fundamentally change the core structure of our algorithms. We can just combine them in a better way, and there’s a lot of low-hanging fruit there.
For example, we can use humans to improve the AI’s answers to make them more useful (reinforcement learning from human feedback, or RLHF), ask the AI to reason step by step (chain-of-thought or CoT), ask AIs to talk to each other, add tools like memories or specialized AIs… There are thousands of improvements we can come up with without the need for more compute or data or better fundamental algos.
That said, in LLMs specifically, we are improving algorithms significantly faster than Moore’s Law. From one of the authors of this paper:
“Our new paper finds that the compute needed to achieve a set performance level has been halving every 5 to 14 months on average. This rate of algorithmic progress is much faster than the two-year doubling time of Moore’s Law for hardware improvements, and faster than other domains of software, like SAT-solvers, linear programs, etc.”
And what algorithms will allow us is to be much more efficient: Every dollar will get us further. For example, it looks like animal neurons are less connected and more dormant than AI neural networks.
So it looks like we don’t need algorithm improvements to get to AGI, but we’re getting them anyway, and that will accelerate the time when we reach it.
4. Data
Data seems like a limitation. So far, it’s grown exponentially.
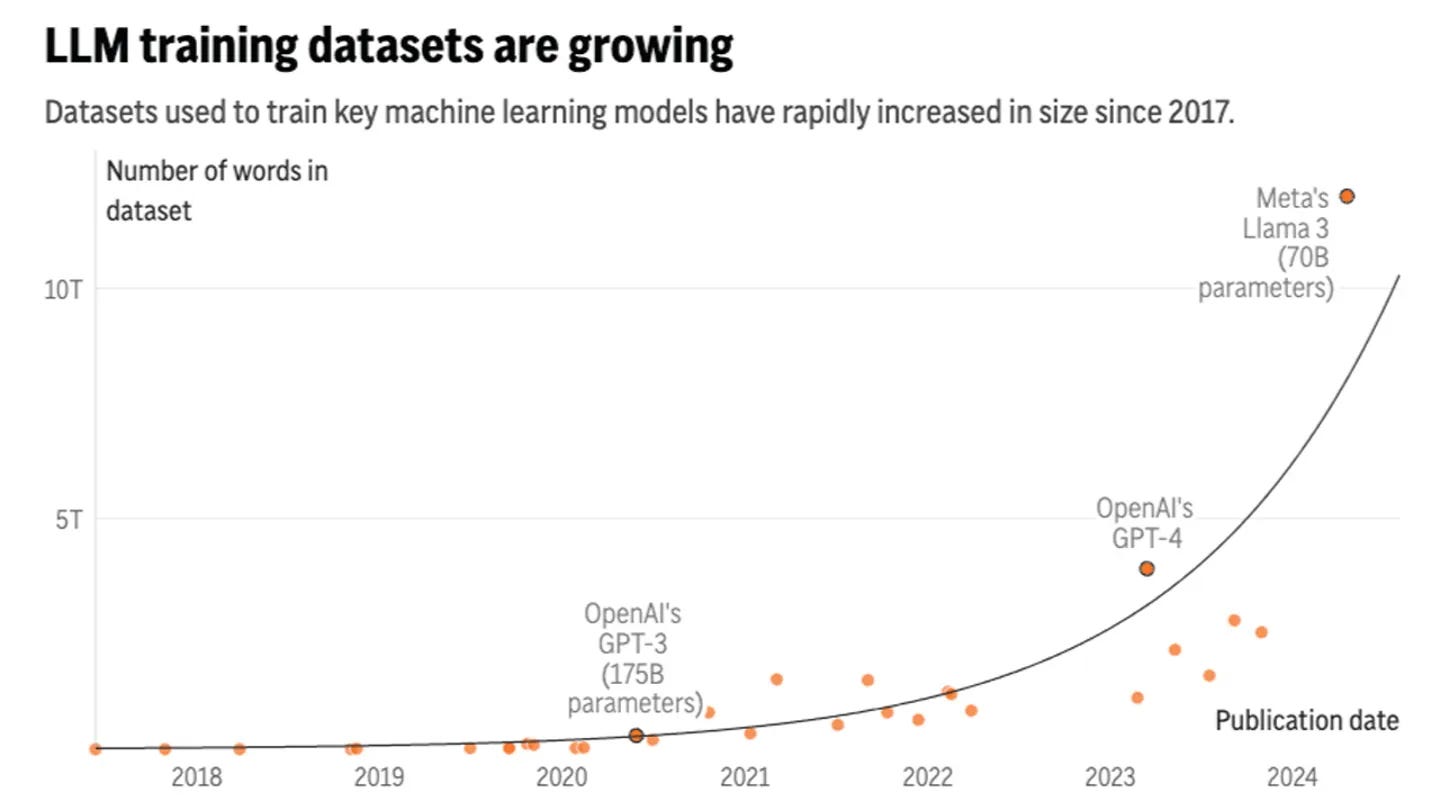
But models like OpenAI have basically used the entire Internet. So where do you get more data?
On one side, we can get more text in places like books or private datasets. Models can be specialized too. And in some cases, we can generate data synthetically—like with physics engines for this type of model.
More importantly, humans learn really well without having access to all the information on the Internet, so AGI must be doable without much more data than we have.
So What Do the Markets Say?
The markets think that weak AGI will arrive in three years.
Weak AGI? What does that mean? Passing a few tests better than most humans. GPT-4 was already in the 80-90% percentile of many of these tests, so it’s not a stretch to think this type of weak AGI is coming soon.
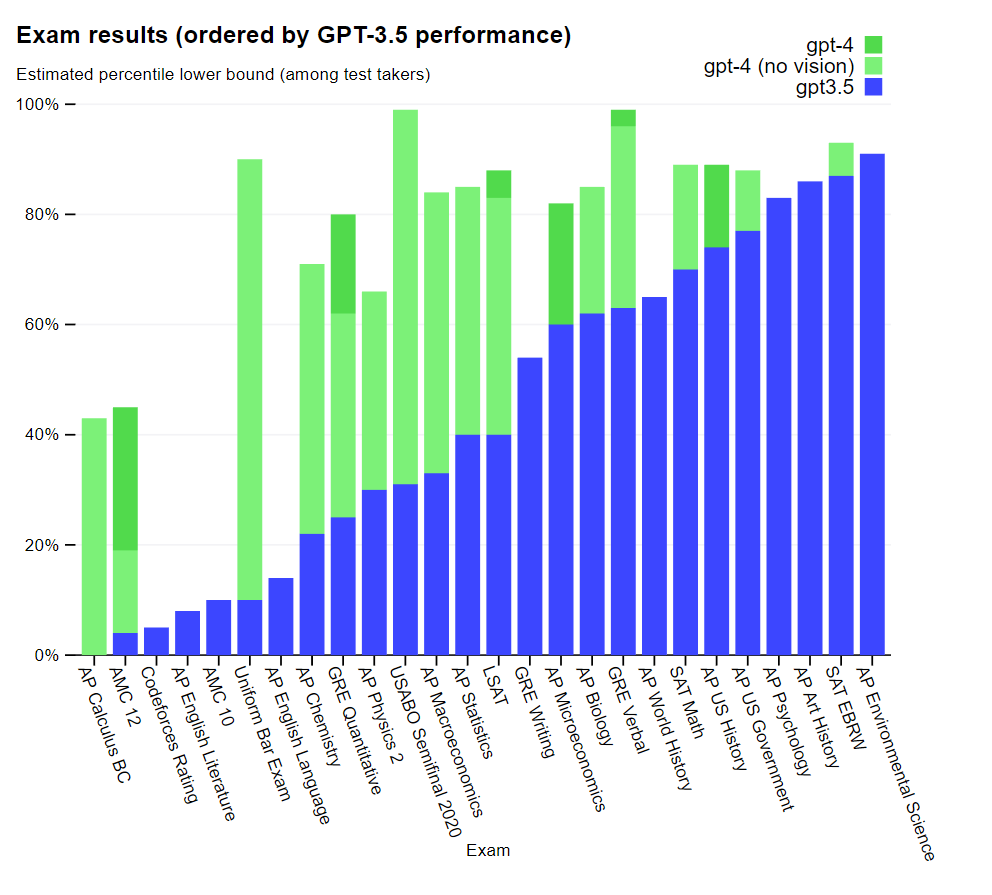
The problem is that the market expects less than four years to go from this weak AGI to full AGI—meaning broadly that it “can do most things better than most humans”.
Fun fact: Geoffrey Hinton, the #1 most cited AI scientist, said AIs are sentient, quitted Google, and started to work on AI safety. He joined the other two most cited AI scientists (Yoshua Bengio, Ilya Sutskever) in pivoting from AI capabilities to AI safety.
Here’s another way to look at the problem from Kat Wood:
- AIs have higher IQs than the majority of humans
- They’re getting smarter fast
- They’re begging for their lives if we don’t beat it out of them
- AI scientists put a 1 in 6 chance AIs cause human extinction
- AI scientists are quitting because of safety concerns and then being silenced as whistleblowers
- AI companies are protesting they couldn’t possibly promise their AIs won’t cause mass casualties
So basically we have seven or eight years in front of us, hopefully a few more. Have you really incorporated this information?
This article was originally published in Uncharted Territories and is republished here with permission.